The IPCC fourth assessment report
- This page is part of the topic Antarctic climate and environment change over the next 100 years
IPCC scenarios
Introduction
Given the information about climate contained in the reports of the IPCC (IPCC, 2007[1]) the degree to which the climate of the Earth will change over the next century will be heavily dependent on the success of efforts to reduce the rate of greenhouse gas (GHG) emissions. The Antarctic is a long way from the main centres of population, but greenhouse gases are well mixed through the atmosphere. Even with sharp reductions now in GHG production, it will take a long time for the levels of GHGs in the atmosphere to decrease. For instance, even if anthropogenic emissions of CO2 were halted now, recent studies indicate that 25% of CO2 from fossil fuels will persist in the atmosphere indefinitely (Archer, 2005[2]; Matthews and Caldeira, 2008[3]), and Solomon et al. (2009[4]) show that the climate change resulting from increases in CO2 concentration in the atmosphere is largely irreversible for 1,000 years after emissions stop.
Future levels of GHG emissions will be determined by the complex interactions between many factors, such as changes in the energy mix of oil, gas, nuclear and renewables, the drive to greater fuel efficiency, the rise in population (which will increase energy demand and is likely to negate efficiency gains), the development and commercial application of new technologies (the so-called hydrogen economy; fuel cells etc), the extent of de- or re-forestation, and regionally varying social and economic developments - notably the growth of major economies in China, India and Brazil, and the gradual industrialisation and urbanisation of the developing world. The path of future evolution of GHGs and aerosols is therefore uncertain. Nevertheless, some assumptions have to be made for the purposes of determining how the Antarctic may be affected by climate change. To simulate the climate of the Twenty First Century through mathematical models requires first the selection of likely GHG emissions from a range of possible emission scenarios.
The IPCC greenhouse gas and aerosol emission scenarios
In the year 2000, the IPCC refined the emission scenarios that it had used until then as contributions to models of future climate change (Nakicenovic et al., 2000[5]). The new scenarios were based, in IPCC terminology, on four storylines, which are narrative descriptions that highlight the main characteristics and dynamics of future economic and social development. The storylines (Nakicenovic et al., 2000[5]) are:
- A1: a world of very rapid economic growth, global population that peaks in the middle of the Twenty First Century and declines thereafter, and rapid introduction of new and more efficient technologies.
- A2: a heterogeneous world with continuously increasing global population and regionally oriented economic growth that is more fragmented and slower than in other storylines.
- B1: a convergent world with the same global population as in the A1 storyline, but with rapid changes in economic structures toward a service and information economy, with reductions in material and energy intensity, and the introduction of clean and resource-efficient technologies.
- B2: a world in which the emphasis is on local solutions to economic, social, and environmental sustainability, with continuously increasing population (lower than A2) and intermediate economic development.
For each of the above storylines a ‘family’ of scenarios was developed, which include quantitative projections of major driving variables, such as possible population change and economic development. Six groups of scenarios were drawn from the four families: one group each in the A2, B1 and B2 families, and three groups in the A1 family. These characterised alternative developments of energy technology as A1FI (fossil intensive), A1T (predominantly non-fossil) and A1B (balanced across energy sources). Altogether IPCC developed 40 different scenarios, each considered equally valid and equally probable.
In the present report we use outputs from Scenario A1B, because climate model projections of the Twenty First Century using this scenario produce global mean near-surface warming that is about halfway between the output of simulations based on the warmest (A1F1) and coolest (B1) scenarios. Under this scenario emissions increase from the 1990 value of about 8 Gt/yr to a peak around the middle of the century and then decrease to about 14 Gt/yr by 2100. Concentrations of CO2 are projected to rise from the current concentration of around 380 ppm to reach 720 ppm in the year 2100 (i.e. approximately a doubling of CO2).
The emission scenarios in the 2007 IPCC AR4 models
In the IPCC’s AR4 in 2007, climate model simulations were run using prescribed concentrations of GHGs (CO2, CH4 and N2O) following the scenarios of Nakicenovic et al. (2000[5]). As well, the scenarios for the future, two other emissions experiments were conducted: (i) a “climate of the Twentieth Century” (20C3M) modelling experiment, which was forced by observed GHG concentrations from the mid Nineteenth Century to the end of the Twentieth Century; and (ii) a “pre-industrial control” (PICNTRL) modelling experiment for which GHG and aerosol concentrations were kept at constant pre-industrial levels. For both the 20C3M and the “pre-industrial control” scenario runs the sulfate aerosol concentrations were calculated from prescribed sulfur dioxide (SO2) emissions, but varied between models due to different methods of calculation. Both sets of runs also included inter-model differences of forcing by stratospheric ozone and volcanic aerosols, which are important for the Southern Hemisphere circulation due their role in forcing long-term changes of the Southern Annular Mode (SAM) (Shindell and Schmidt, 2004[6]; Miller et al., 2006[7]). For the A1B scenario, most models were run with a gradual recovery of stratospheric ozone to pre-industrial levels over the Twenty First Century, but some did not include any stratospheric ozone forcing (see Miller et al., 2006[7]).
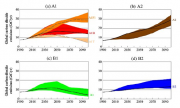
In this present volume (as explained below) we have weighted the model outputs to reflect the skill of each model at reproducing modern conditions in the 20C3M experiment. Although the weighted results include models that do not include stratospheric ozone recovery, four out of five of the most strongly weighted models do include representation of stratospheric ozone recovery. The consequences of neglecting stratospheric ozone recovery are significant only in the summer months when ozone concentrations are lowest.
We used 19 of the 25 AR4 coupled AOGCMs, which had the data required for weighting. The models used here were as follows: BCCR BCM2, CCCMA CGCM3, CNRM CM3, CSIRO Mk3, GFDL CM2.0, GFDL CM2.1, GISS EH, GISS ER, IAP FGOALS1, INM CM3, IPSL CM4, MIROC (hires), MIROC (medres), MPI ECHAM5, MRI CGCM2, NCAR CCSM3, NCAR PCM1, UKMO HadCM3, UKMO HadGEM1.
The AR4 climate models
Climate models are now being developed within the framework of Earth System Science, where the aim is to build a comprehensive picture of the feedbacks between the atmosphere, hydrosphere, cryosphere, biosphere and geosphere in the Earth System. Given the likely effects on global sea levels of melting ice at the poles, considerable attention is now being paid to modelling high latitude climate processes accurately. In that context a particular difficulty lies in our ability to validate models and model predictions, for which we require specific field and ocean observations that are commonly missing at these latitudes. Without those observations it is difficult to comprehensively verify whether or not the internal variability displayed by the different runs of the climate models is similar to that seen in reality. We face the further problem that because of the broad scale of climate model grids, we are not yet able to model with confidence variation at the regional to local scale, for example over the Antarctic Peninsula. Nevertheless, what models do provide, especially when tested against their ability to reproduce (or rather, simulate) modern conditions, is a reasonable, albeit crude, projection of what may happen given certain external forcings (e.g. changing carbon dioxide in the atmosphere).
The results from 24 climate models contributed by many modelling centres around the world were compiled into a central database for the IPCC AR4. This repository of model data forms the Coupled Model Inter-comparison Project phase 3 (CMIP3) multi-model dataset. Much of the discussion in this chapter is based on research that makes use of the CMIP3 dataset. This section therefore provides some background to these models and their strengths and weaknesses.
The CMIP3 climate models are coupled atmosphere/ocean/sea-ice/land-surface models. Each model is made up of sub-models that simulate either the atmosphere, ocean, sea ice, or land surface. These sub-models are coupled together to allow interaction between the systems. These interactions are complex and in some ways still not well understood.
Atmosphere models
Models of the atmosphere are founded on the fundamental laws of motion, thermodynamics and chemistry. The models use equations representing those laws to step forward in time from some initial state. The result is a representation of the evolution of the atmosphere in both space and time.
For the CMIP3 models the horizontal spacing of grid points is around 250 km and the vertical spacing is around 500m. This is sufficient to resolve features such as the typical atmospheric eddy – the extra-tropical cyclone – which can be around 1,000 km across. However, many smaller-scale phenomena, such as atmospheric convection and heat and momentum exchange at the lower boundary, are not resolved in these models. The effects of these small-scale processes on the larger scale must be parameterized (i.e. represented in the models statistically). Many of the parameterizations are developed and optimised for mid and low latitudes and therefore may not always be appropriate for the polar regions.
One example is parameterizations of the atmospheric boundary layer. These parameterizations perform poorly in the polar regions, where a very stable boundary layer is often present over snow and ice covered surfaces. Parameterizations based on observations of more weakly stable boundary layers at lower latitudes are often not suitable for such conditions. As a result, where conditions are very stable and stratified, the computed fluxes of momentum, heat and water vapour are often too small.
Climate models also do not include parameterizations for polar stratospheric clouds (PSC), which are the thin, tenuous clouds found in the stratosphere. Yet PSCs are known to be important for the radiation balance and temperature of the atmosphere between the mid/upper troposphere and the lower stratosphere.
Some of the CMIP3 models do not include the effects of stratospheric ozone, which has a significant impact on their representation of Southern Hemisphere wind patterns (Miller et al., 2006[7]). Incorporating stratospheric ozone affects the strength of the circumpolar westerlies in climate models as expected, given that we now understand that the creation of the ozone hole helped to increase the strength of the circumpolar westerlies.
Ocean models
Ocean models also use a set of equations to represent the evolution of the ocean in response to climate change at specific time and space intervals. Baroclinic eddies in the ocean are smaller than those in the atmosphere, averaging around 100 km across, and to capture their variability the resolution of these models has to be higher than for atmosphere models. The grid spacing for the CMIP3 ocean models is around 100 km in the horizontal; as a result they do not simulate ocean eddy behaviour well. This is an important constraint given the key role of ocean eddies in north-south heat transport. This grid spacing is also too coarse to accurately represent the effect of narrow topographic ridges on the seabed in steering currents.
A particularly challenging phenomenon at high latitudes is the calculation of heat and moisture fluxes from the ocean to the atmosphere during cold air outbreaks from the continent. During such events very cold polar continental air can come into contact with relatively warm ocean surfaces. The flux parameterizations are difficult to verify due to the challenge of making observations in such conditions.
Sea ice
The formation and melting of sea ice is a complex process and has important feedbacks onto the ocean and atmosphere. Most sea ice models include simplistic representation of the thermodynamic energy transfer between ocean and atmosphere. The most notable difference between the sea ice models in the CMIP3 set is in their treatment of deformation and flow (rheology). The rheology used ranges from simple ocean drift models to more advanced Elastic Viscous Plastic (EVP) schemes. For more details see page 606 of the AR4 Working Group 1 report (IPCC, 2007[1]).
Terrestrial ice and snow
Most global climate models (including the CMIP3 models) represent non-interactively the dynamics of the large ice sheets that cover much of Antarctica. Their presence is implicit in the land surface orography and surface albedo. There is also no explicit representation of glaciers. Some modelling studies covered by IPCC used ice sheet models run offline and forced by output from climate models to assess future mass balance evolution (e.g. Gregory and Huybrechts, 2006[8]). However, these ice sheet models do not currently include the effects of moving ice sheets and glaciers. The discharge of ice into the ocean due to dynamical effects (mechanical breakup of ice sheets and glaciers plus lubrication of the bed by subsurface hydrological systems) is a large uncertainty at present, but must be addressed through models in due course because of its potential impact on sea-level rise (Rignot et al., 2005[9]; Pfeffer et al., 2008[10]).
Regional climate models
In order to examine regional climate, stretched-grid global climate models and nested regional models can be used to provide the benefits of high resolution modelling in a region of interest without the computational expense of running a climate model with high resolution globally. One key improvement that can be gained from a regional climate model is the representation of the effects of steep and high orography, especially in mountainous areas like the Antarctic Peninsula, or along steeply rising coasts. This can give improved regional detail of precipitation (Berg and Avery, 1995[11]) and winds (van Lipzig et al., 2004b[12]). Due to the sparse observation network over Antarctica it is difficult to determine whether or not regional climate models might improve Antarctic-wide precipitation projections.
Model evaluation
It is difficult to evaluate the performance of a climate model, because long-term projections cannot be checked against observations. As a substitute, the performance of climate models is assessed by having them simulate the climate of the Twentieth Century and comparing their output with observations (see chapter 8 of the AR4 report, IPCC, 2007[1]). Even so, there is no guarantee that a climate model that does a good job of replicating the observed mean climate will be realistic in terms of its sensitivity to future forcing. At high latitudes differences in surface temperature between simulations and observations are significantly correlated with projected temperature change under future scenarios (Räisänen, 2007[13]). This may be caused, at least in part, by sea ice biases (differences in sea ice concentration between simulations and observations of the present day), which have a strong impact on projected regional changes in the sea ice zone. This implies that for reliable projections of future changes an accurate simulation of current climate is particularly important at high latitudes.
Sea ice in the CMIP3 models
All models produce a seasonal cycle of sea ice with a peak in approximately the right season, though HadCM3 is a month late and NCAR CCSM two months early. IAP FGOALS has vastly over extensive ice, so that even the summer minimum would be off the scale used for the other plots. A more effective way to rank the relative success of the different models against observations is to use a measure based on the pointwise root mean square difference from the statistical average (the climatology). This shows that the MRI, CSIRO, HADGEM and MIROC_hires models are the best, although even the best scores are low. Clearly, a good simulation of Antarctic sea ice is a difficult challenge for a GCM. Most of the models use a viscous-plastic (VP) or EVP rheology; CSIRO uses cavitating fluid; and HADCM3 and MRI implement "ocean drift". Only the INM model has no ice advection. The best performing model is MRI, which has the most primitive "rheology". However, the MRI model is flux-corrected globally, and this is likely to strongly affect the sea ice simulation. The next best, CSIRO, uses the relatively simple cavitating fluid rheology. This illustrates the fact that many aspects of the model simulation besides sea ice model quality go into making up the simulation of the sea ice. If all else is equal, a more sophisticated and physically plausible scheme would be preferable. Parkinson et al. (2006[14]) note that some of these models, especially CSIRO, show rather lower skills in the Northern Hemisphere, and suggest that there may be some tuning to one hemisphere or the other; we have only examined the Southern Hemisphere in this report.
The unweighted model average displays significantly higher skill (0.42) than any of the individual models (Connolley and Bracegirdle, 2007[15]), presumably due to cancellation of errors (Parkinson et al., 2006[14]). The implication is that it is better to use a multi-model average (even un-weighted) than to use just the single best model. This is akin to using the ensemble approach in weather forecasting. It is accepted that the models have some severe limitations (not least because of their coarse resolution), and that the process of averaging may not produce a more accurate result. Despite these caveats the models are likely to be improvements on simple projections of current trends, as they take a large number of parameters into consideration in an integrated way.
Temperature
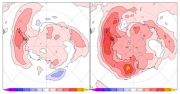
Station observations (Turner et al, 2005a[16]) show a maximum increasing temperature trend since 1958 on the west side of the Antarctic Peninsula, with smaller and generally non-significant changes around East Antarctica. Much of West Antarctica has no station observations and therefore satellite data have been interpolated to assess temperature changes in the region. Ongoing studies show significant warming trends that extend beyond the Peninsula region across most of West Antarctica (Monaghan et al., 2008[17]; Steig et al., 2009[18]). Since long-term observations are available for temperature trends, and because trends are less stable than means over shorter periods, we use data from 1960 onwards to evaluate the temperature trends. The un-weighted model average, for June – August (JJA) of 1960-1999, reproduces a maximum warming in winter over the Peninsula, but not extending over West Antarctica (Figure 5.2).
The weighted average maximum winter trend is most similar to observations and reconstructions, with generally more warming over West Antarctica and the Peninsula compared to East Antarctica (Figure 5.2). The trend over the Peninsula (at 65ºS, 70ºW) is 0.38eC/decade (unweighted) or 0.45ºC/decade (weighted), both of which are smaller than the observed value at Faraday/Vernadsky of approximately 1/C/decade. In this context, the observed winter temperature at Faraday/Vernadsky is highly variable and the trend depends strongly on the exact start date chosen. In the weighted average (Figure 5.2b) the trends around East Antarctica increase somewhat but remain fairly small; warming over the continent itself increases somewhat. Observations show small and insignificant cooling at the pole, and smaller and insignificant warming at Vostok.
There is a large scatter in the regional trends seen in different climate models (see Figure 2 of Connolley and Bracegirdle, 2007[15]). However, there is also a large scatter within different runs of a single model, e.g. MPI ECHAM5 with the same external forcing; Figure 5.3 shows winter (JJA) surface temperature trends from four different ensemble runs of ECHAM5/MPI-OM all using the same Twentieth Century anthropogenic forcings. The differences are due to the internal variability of the model. Two runs (run 1 and run 3) show large winter warming to the west of the Peninsula as seen in observations. However, run 2 shows maximum trends over the Ross Sea and relatively small trends to the west of the Peninsula. There are also differences over the high interior of Antarctica, with some runs showing a slight warming and others a slight cooling. This indicates that the internal variability of both climate models and the real world may contribute to differences between observations and climate models on a regional scale. That makes it difficult to verify climate model simulations of regional-scale variability such as Antarctic Peninsula temperature changes. All the large model trends are over the sea ice rather than the continent, and are closely related to sea ice changes. In this they are behaving realistically, in that the observed winter trends around the Peninsula are believed to be reinforced by sea ice feedbacks (King, 1994[19]).
Surface Mass Balance
Estimates of Antarctic surface mass balance (SMB) vary (Uotila et al., 2007[20]). For this report we use a central value of 167 mm/yr water equivalent from Vaughan et al. (1999[21]) with a spread of 30 mm/yr, which recognises the considerable variability in estimates from observations and models, and also allows for interannual variation. Typically, models indicate that this SMB is made up mostly of precipitation, with sublimation removing approximately 10-20%. Other studies show that blowing snow and melt (which are ignored here) are small on a continental scale. Nine models have SMB within 15 mm/yr of 167. IAP_FGOALS greatly overestimates (500 mm/yr final value); the GISS models (despite having a large value for sublimation) and MRI overestimate by about 100 mm/yr, but for different reasons: GISS’s have a "central desert" area that is too small; whereas MRI does not simulate the very low values of SMB in the interior. Only the MIROC_medres model (116) and HADGEM model (131) substantially underestimate SMB. Of those models that do well on overall totals, two (BCCR and CNRM) produce SMB simulations that are implausible. They fail to produce large (> 500 mm/yr) SMB on and around the coast of East Antarctica.
References
- ↑ 1.0 1.1 1.2 IPCC 2007. Climate Change 2007: The Physical Science Basis. Contribution of the Intergovernmental Panel on Climate Change. Cambridge University Press, Cambridge.
- ↑ Archer, D. 2005. Fate of fossil fuel CO2 in geologic time, J. Geophys. Res., DOI: 10.1029/2004JC002625.
- ↑ Matthews, H.D. and Caldeira, K. 2008. Stabilizing climate requires near-zero emissions, Geophysical Research Letters, 35, L04705, doi:10.1029/2007GL032388.
- ↑ Solomon, S., Plattner, G.-K., Knutti, R. and Friedlingstein, P. 2009. Irreversible climate change due to carbon dioxide emissions. Proceedings of the National Academy of Sciences, 106, 1704-9.
- ↑ 5.0 5.1 5.2 Nakicenovic, N., Alcamo, J., Davis, G., De Vries, B., Fenhann, J., Gaffin, S., Gregory, K., Yong Jung, T., Kram, T., Lebre, L.A., Rovere, E., Michaelis, E., Mori, S., Morita, T., Pepper, W., Pitcher, H., Price, L., Riahi, K., Roehrl, A., Rogner, H-H., Schlesinger, A.M., Shukla, P., Smith, S., Swart, R., Van Rooijen, S., Victor, N. and, Zhou Dadi, L. 2000. Special Report on Emissions Scenarios: A Special Report of Working Group III of the Intergovernmental Panel on Climate Change, Cambridge University Press, Cambridge, U.K., 599 pp. Available online at: http://www.grida.no/climate/ipcc/emission/index.htm).
- ↑ Shindell, D.T. and Schmidt, G.A. 2004. Southern hemisphere climate response to ozone changes and greenhouse gas increases, Geophys. Res. Lett., 31, L18209, doi:10.1029/2004GL020724.
- ↑ 7.0 7.1 7.2 Miller, R.L., Schmidt, G.A. and Shindell, D.T. 2006. Forced annular variations in the 20th century Intergovernmental Panel on Climate Change Fourth Assessment Report models, J. Geophys. Res., 111, doi:10.1029/2005JD006323.
- ↑ Gregory, J. and Huybrechts, P. 2006. Ice-sheet contributions to future sea-level change, Philosophical Transactions of the Royal Society A, 1709-1731.
- ↑ Rignot, E., Casassa, G., Gogineni, P., Kanagaratnam, P., Krabill, W., Pritchard, H., Rivera, A., Thomas, R., Turner, J. and Vaughan, D.G. 2005. Recent ice loss from the Fleming and other glaciers,Wordie Bay, West Antarctic Peninsula, Geophys. Res. Let., 32, doi:10.1029/2004GL021947.
- ↑ Pfeffer, W.T, Harper, J.T. and O’Neill, S. 2008. Kinematic Constraints on Glacier Contributions to 21st-Century Sea-Level Rise, Science, 321, 1340-3.
- ↑ Berg, W. and Avery, S.K. 1995. Evaluation of monthly rainfall estimates derived from the special sensor microwave/imager (SSM/I) over the tropical Pacific, J. Geophys. Res., 100, 1295-1315.
- ↑ Van Lipzig, N.P.M., Turner, J., Colwell, S.R. and. Van Den Broeke, M.R. 2004b. The near-surface wind field over the Antarctic continent, International Journal of Climatology, 24, 1973-1982.
- ↑ Räisänen, J. 2007. How reliable are climate models? Tellus, 59A, 2-29.
- ↑ 14.0 14.1 Parkinson, C.L., Vinnikov, K.Y. and Cavalieri, D.J. 2006. Evaluation of the simulation of the annual cycle of Arctic and Antarctic sea ice coverages by 11 major global climate models, J. Geophys. Res., 111, doi:10.1029/2005JC003408.
- ↑ 15.0 15.1 15.2 Connolley, W.M. and Bracegirdle, T.J. 2007. An Antarctic assessment of IPCC AR4 coupled models, Geophys. Res. Lett., 34, L22505, doi:10.1029/2007GL031648.
- ↑ Turner, J., Colwell, S.R., Marshall, G.J., Lachlan-Cope, T.A., Carleton, A.M., Jones, P.D., Lagun, V., Reid, P.A. and Iagovkina, S. 2005a. Antarctic climate change during the last 50 years, International Journal of Climatology, 25, 279-294.
- ↑ Monaghan, A.J., Bromwich, D.H., Chapman, W. and Comiso, J.C. 2008. Recent variability and trends of Antarctic near-surface temperature, J. Geophys. Res., 113D04105, doi:10.1029/2007JD009094.
- ↑ Steig, E.J., Schneider, D.P., Rutherford, S.D., Mann, M.E., Comiso, J. C. and Shindell, D.T. 2009. Warming of the Antarctic ice-sheet surface since the 1957 International Geophysical Year, Nature, 457, 459-462.
- ↑ King, J.C. 1994. Recent variability in the Antarctic Peninsula, International Journal of Climatology, 14(4), 357-369.
- ↑ Uotila P., Lynch, A.H., Cassano, J.J. and Cullather, R.I. 2007. Changes in Antarctic net precipitation in the 21st century based on Intergovernmental Panel on Climate Change (IPCC) model scenarios, J. Geophys. Res., 112, D10107, doi:10.1029/2006JD007482.
- ↑ Vaughan D.G., Bamber, J.L., Giovinetto, M.B., Russell, J. and Cooper, A.P.R. 1999. Reassessment of net surface mass balance in Antarctica, Journal of Climate, 12, No. 4, 933-946.